Chip发表中南大学、复旦大学最新合作成果:面向高效神经形态计算的范德华铁电晶体管全能人工突触
FUTURE远见| 2023-06-02
Future|远见
Future|远见future选编
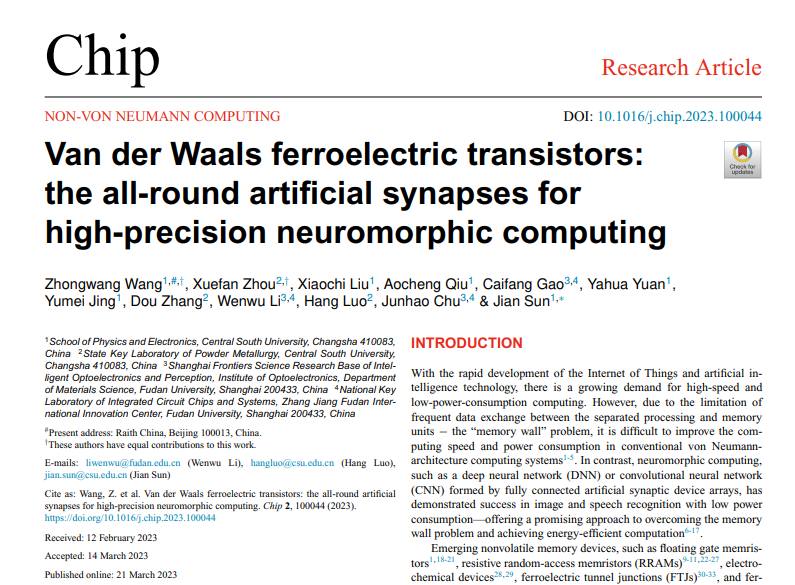
大数据时代对高速、高密度、高带宽、低功耗的高性能计算来处理海量数据的需求呈爆发式增长,然而高性能计算越来越受限于逻辑单元与存储单元的数据传输瓶颈。基于人工突触器件阵列神经网络的神经形态计算在低功耗图像和语音识别方面表现除了强大的应用潜力,为克服「内存墙问题」和实现低功耗高效计算提供了重要的技术路线。在众多面向神经形态计算硬件的候选器件中,铁电晶体管(FeFET)由于其简单的器件结构,非易失性的数据存储时间、非破坏性的读取、纳秒级的编程/擦除速度、低电压/功耗的操作等技术优势而受到关注。然而,以铪基铁电薄膜和硅等薄膜材料开发铁电晶体管需要在界面引入缓冲界面层来防止两者之间的化学反应,不仅浪费功耗,还会引发界面问题。此外薄膜界面处还存在由于晶格失配、应变、缺陷等不利因素会造成的复杂的界面因素²,影响器件性能,造成反复擦写能力较差、部分极化状态不稳定等缺点。
二维材料表面没有悬挂键,通过范德华堆叠技术构建异质结,避免了薄膜生长过程中的诸多不利因素,可以与铁电材料形成高质量的范德华界面,用于优化界面问题导致的高功耗、退极化场等问题。最近,人们利用铁电材料,例如HfZrO₂,LiNbO₃,P(VDF-TrFE),CuInP₂S₆与二维半导体集成,构筑了混合维度范德华异质机构铁电晶体管(vdW-FeFET)并拓展了它们在神经形态计算领域的应用。高效的神经形态计算需要人工突触器件满足几个关键的突触特性,包括高线性度和对称的电导增强/抑制权重更新,大于100的导电态、低于5 V的低操作电压和大于100的动态范围。基本上没有报道的FeFET可以同时满足所有要求。其中低线性度的权重更新特性是造成神经网络低识别精度的主要原因,限制了基于FeFET的神经形态计算硬件发展。研究人员可以通过设计非相同递增脉冲方案改善了铁电晶体管突触权重更新的线性度和对称性。在FeFET中同时满足各项高效神经形态计算所需要的器件指标仍然是一个技术难题。
在这项研究中,研究团队利用范德华异质集成技术构筑了由单晶铁电纳米片、二维半导体通道和石墨烯范德华接触组成的全范德华结构vdW-FeFET人工突触器件(图1)。研究利用压电力探针技术展示了铁电单晶的多电畴结构在相同电压脉冲序列控制下极化逐步翻转的特性。
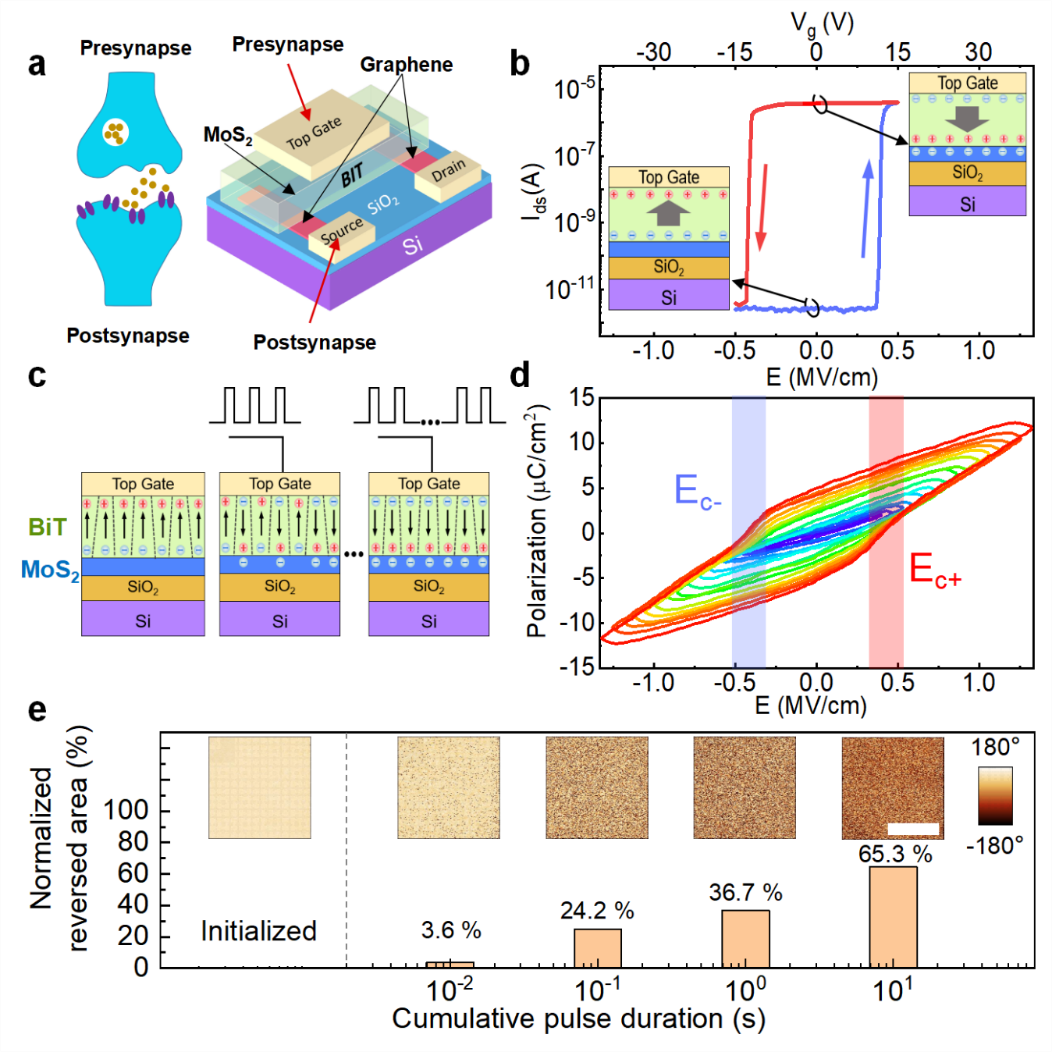
在相同栅极脉冲方案的调控下,vdW-FeFET表现出了极高线性(增强~0.016,抑制 ~0.013)和高对称性(0.003)的权重更新特性。研究团队在vdW-FeFET中展示了5个电导态的长达10年的存储非易失性以及大于100个周期的稳定操作。vdW-FeFET同时展示了其他关键的人工突触器件性能,即大于128个电导状态、小于10 fJ/脉冲的低操作功耗,小于5 V的操作电压。研究团队基于vdW-FeFET器件性能模拟了双层神经网络对于手写数字和心电图模式的识别(图2),分别获得了97.6%和92.4%的高精度。vdW-FeFET人工突触器件在各项器件指标都表现出了良好的均衡性能,是面向高精度神经形态计算的潜在硬件平台。
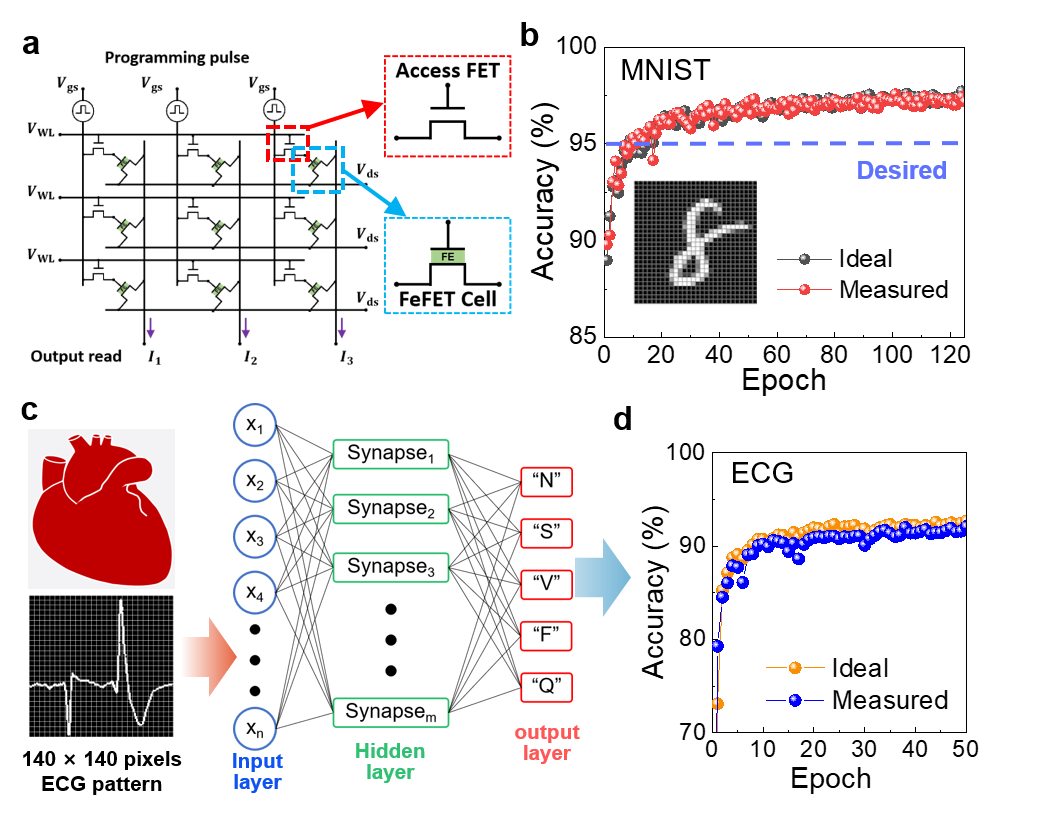
Van der Waals ferroelectric transistors: the all-round artificial synapses for high-precision neuromorphic computing¹
Neuromorphic computing with the neural network formed by fully connected artificial synaptic device arrays, has demonstrated success in image and speech recognition with low power consumption—offering a promising approach to overcoming the memory wall problem and achieving energy-efficient computation. Emerging nonvolatile memory devices with programmable multi-state memory characteristics are promising hardware candidates to achieve neuromorphic computation. FeFETs have drawn attention because of their fast operation, high conductance tunability, long retention, and low operating power. Thin-film-based FeFETs typically suffer from complex interfacial issues, including interfacial layers and charge traps, caused by the lattice mismatch or chemical reaction between ferroelectric and semiconductor crystals, resulting in performance degradation and an increase in power consumption². Two-dimensional (2D) semiconductors with an atomically thin nature and dangling bond-free surfaces can be stacked with ferroelectrics via van der Waals (vdW) forces to overcome interfacial problems.
To date, various ferroelectric materials have been integrated with 2D semiconductors to build mixed-dimensional or vdW-FeFETs for neural networks. As known that, state number, operation power, dynamic range, and conductance weight update linearity are critical synaptic device performance metrics for high-accuracy, low-power-consumption neuromorphic computing in hardware³. However, most of the reported synaptic devices cannot simultaneously achieve high linearity and low power consumption, limiting hardware performance. In this work, the researchers demonstrated van der Waals (vdW) stacked ferroelectric field-effect transistors (FeFET) with single-crystalline ferroelectric nanoflakes. Because of the fine vdW interface and the partial polarization switching of multi-domains in ferroelectrics under electric field pulses, the vdW-FeFETs exhibit multi-state memory characteristics and excellent synaptic plasticity. These devices also exhibit a desired linear conductance weight update with 128 conductance states, sufficiently high dynamic range >120, and low power consumption of 10 fJ/spike using identical pulses. Based on such an all-round device, the researchers built a two-layer artificial neural network to conduct Modified National Institute of Standards and Technology digital numbers and electrocardiogram pattern-recognition simulations, with high accuracies reaching 97.6% and 92.4%, respectively. The remarkable performance highlights the advantage of vdW-FeFET for high-precision neuromorphic computing applications.
参考文献:
1. Wang, Z. et al. Van der Waals ferroelectric transistors: the all-round artificial synapses for high-precision neuromorphic computing. Chip 2, 100044 (2023).
2. Mulaosmanovic, H., Breyer, E. T., Dünkel, S., Beyer, S., Mikolajick, T. & Slesazeck, S. Ferroelectric field-effect transistors based on HfO₂: a review. Nanotechnology 32, 502002 (2021).
3. Yu, S. Neuro-inspired computing with emerging nonvolatile memorys. Proc. IEEE 106, 260-285 (2018).
文章链接:
https://www.sciencedirect.com/science/article/pii/S2709472323000072
关于Chip
Chip是全球唯一聚焦芯片类研究的综合性国际期刊,已入选由中国科协、教育部、科技部、中科院等单位联合实施的「中国科技期刊卓越行动计划高起点新刊项目」,为科技部鼓励发表「三类高质量论文」期刊之一。
Chip期刊由上海交通大学与Elsevier集团合作出版,并与多家国内外知名学术组织展开合作,为学术会议提供高质量交流平台。
Chip秉承创刊理念: All About Chip,聚焦芯片,兼容并包,旨在发表与芯片相关的各科研领域尖端突破性成果,助力未来芯片科技发展。迄今为止,Chip已在其编委会汇集了来自14个国家的70名世界知名专家学者,其中包括多名中外院士及IEEE、ACM、Optica等知名国际学会终身会士(Fellow)。
Chip第二卷第二期(2023年夏刊)即将于2023年6月在爱思维尔Chip官网以金色开放获取形式(Gold Open Access)发布,欢迎访问阅读本期最新文章。
爱思唯尔Chip官网:
https://www.journals.elsevier.com/chip